On systemic health indicators (part 2)
In part 1 of this series we saw how the ‘state of a system’ can be seen as a ‘marble’ at a certain position in a ‘stability landscape’.
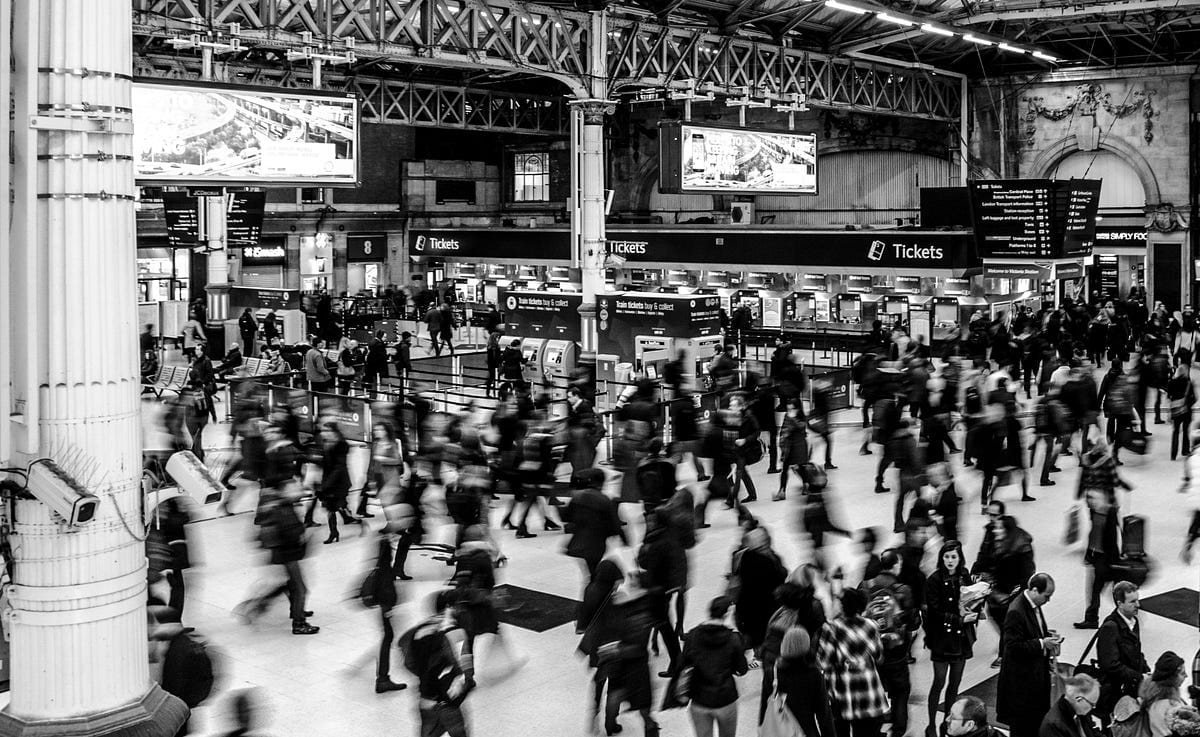
In part 1 of this series we saw how the ‘state of a system’ can be seen as a ‘marble’ at a certain position in a ‘stability landscape’.
In this section, we look at how this can translate into health indicators.
“Forces” (from inside or outside the system) can push this marble from one valley into another valley. But the landscape itself can change as well. A valley can become a mountain, or a trench can materialize, for example. In the case of a human system, examples of a changing landscape are: an accident or illness, a change in diet, or in exercising behavior. Those are outside forces. Regular growth can be seen as a force from within the system that changes the landscape.
This applies to any ‘system’, on any scale, whether it be a bacterium, a human body, a forest, an economic system, a financial system, etc. Ultimately, our socio-economic / financial domains (systems) are the most interesting, especially in the context of problems we experience with the impact of globalization and technological progress on our living environment and social cohesion.
Diagnostics
The idea is to use this stability landscape to read ‘systemic health aspects’: we can use it as a ‘diagnostic tool’. We can then determine what a desired ‘intervention’ would be and monitor whether it has the desired effect.
In fact, this is exactly what politicians already do: they look at socio-economic and other aspects, and formulate a policy based on these aspects. Also, it looks like what a doctor already does. But now, with these landscapes, we are looking at a much higher level of abstraction, so high that it actually applies to most systems. At this abstraction level, it will get a bit more complicated at first, but then a lot more powerful.
As an aside, I suspect that a number of current socio-economic developments would look very similar in such an analysis, even though they appear very different in the real world. You will not immediately see the growing difference between rich and poor in a changing landscape, but it will be related to a specific change in that landscape, like many other symptoms.
That said, steering on systemic health indicators in stability landscapes is currently completely unknown territory, especially for systems such as our socio-economic and financial domains.
A roadmap
In practical terms, we could build this new ‘discipline’ in 4 learning steps:
- being able to determine the landscape, and the position of the systemic state in this landscape (or its trend) (quantitative system analysis)
- being able to determine which places, aspects and trends of the landscape are ‘healthy’ and ‘unhealthy’ (desirable/undesirable) (qualitative system analysis)
- understand how we can move the systemic state within the landscape (pushing the marble to a ‘healthier’ place) (state intervention)
- understand how we can alter the landscape (landscape intervention)
The difficulty — abstraction
In order to determine the landscapes in a meaningful way, we really need to understand the underlying dynamics, at the most abstract level possible. In many cases, that will be the maximization of entropy production, on a given infrastructure. That is very technical, and I have written on this extensively, but it is, roughly speaking, about saturation processes. You can think of growing markets of mobile phones, the increase of digital information, concentration of capital, etc.
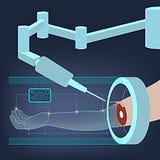
An example: making something more efficient through technology does not necessarily mean that total energy consumption is decreasing, because there will often occur an increase of it occurence as well, because it has become cheaper. And in the digital age, these trends can go very fast, and even go too far. But ultimately the whole system wants to use as much energy as possible in total, so it will be optimized. This is typically an example of such maximization.
(By the way: ‘energy-use’ should be interpreted broadly, here.)
It is therefore about getting the right aspects, and at the right level of abstraction, that is to do with this energy management. They are not always trivial. Think, for example, of financial markets, and how many connections it has with the real world: what kind of energy is actually dissipating over there, and on which infrastructures?
Systemic health indicators
Only after we have completed these steps can we look at health indicators. Both aspects and trends can serve as an indicator.
Some indicators will be domain specific, others will be general. For example, suppose that the state of one of our subsystems is in a valley that we experience as ‘desired’, and that this valley becomes less deep, or smaller in size. Then that clearly indicates a decrease in the stability of that state and that can be unhealthy: the ‘state marble’ can roll out more easily, to a less favorable valley, for example.
Or that a valley splits into several small valleys; perhaps this produces ‘turbulence’ in the system.
An inventory can be made (per domain) of all these aspects (even a taxonomy). It is conceivable that only a small number of types of landscape changes occur in many of our domains. Similar to DSM-V in psychiatry, for example, or what a general practitioner uses for diagnostic methodology. We can see the position of the state of the domain in the landscape (the marble) as a ‘representation of the condition’, and the landscape as a ‘representation of the immune system’.
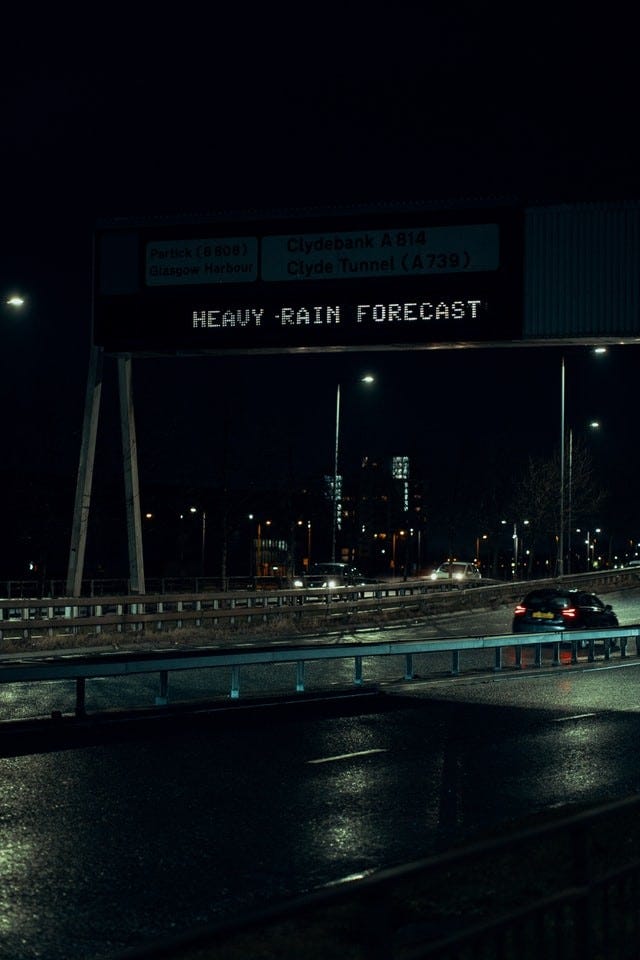
‘Weather forecasts’ for our socio-economic domains
Mankind has managed to crack the codes of our DNA through CRISPR, and to crack financial exchanges through High Frequency Trading, and almost in making the first quantum computer. That is systemic control at micro level.
I see no reason why we can’t crack the codes of macro-level systemic control. It might be a matter of a shift in resource allocation. It seems to me that systemic control is more useful today than some other CRISPR-technology.
In the next part of this series, I will try to jumpstart the four steps mentioned.
In deel 3 zal ik een aanzet geven tot deze 4 stappen.